Running Applications¶
Deploy an Example App¶
Note
This tutorial will show you the pieces required to deploy an example bot to the Rasa Platform.
Goal¶
We will deploy one of the example bots from the Rasa Core repo to your running instance of the
platform.
The easiest way to follow along is if you carry out these steps while in an ssh
session on
the server where you deployed the platform. In the final section we discuss how to build and
deploy to a remote server.
There are two main steps to deploying a bot to the Rasa Platform:
- Creating a docker container where all your actions will be executed
- Making models available to the platform’s Rasa Core and Rasa NLU containers.
Creating an Application and a Docker Container¶
If you haven’t done so already, ssh
into your server.
Clone the demo app:
git clone https://github.com/RasaHQ/platform-demo.git
cd platform-demo/moodbot
Have a look at the file main.py
.
This example app uses the RasaChatInput
. you can find more information
on that here: Rasa Chat.
Running your bot on the platform makes use of a class RemoteAgent
.
import logging
import os
from rasa_core.channels.rest import HttpInputChannel
from rasa_core.remote import RemoteAgent
if __name__ == "__main__":
logging.basicConfig(level="DEBUG")
# instantiate the input channel you want to connect to
from rasa_extensions.core.channels.rasa_chat import RasaChatInput
input_channel = HttpInputChannel(
5001, "/", RasaChatInput(os.environ.get("RASA_API_ENDPOINT_URL")))
agent = RemoteAgent.load('models/dialogue',
os.environ.get("RASA_REMOTE_CORE_ENDPOINT_URL"),
os.environ.get("RASA_CORE_TOKEN"))
agent.handle_channel(input_channel)
Note
To run this application locally or to build it on a CI server, you need an
account on https://pypi.rasa.ai
as this will allow you to fetch the
enterprise versions of Rasa (the rasa_extensions
package).
More information can be found in Python package installation.
Building Your Docker Image¶
To build a docker image which will execute the bot’s actions, run:
docker build -t $IMAGE_TAG --build-arg RASA_PYPI_USER=user --build-arg RASA_PYPI_PASSWORD=pw .
This will use the Dockerfile in the moodbot directory.
For now, you can use something like IMAGE_TAG=demobot:v1
.
If you are deploying to a remote server, you will want to push the image to a registry first.
An image tag like IMAGE_TAG=username/demobot:v1
will allow you to push this tag to a docker registry like GCR or docker Hub.
You can read more about docker tags here: https://docs.docker.com/engine/reference/commandline/tag/
Start the application¶
Once your docker image is built, you can start the container using a command like the below.
This will copy your trained dialogue model to the directory where Rasa Core can find it,
and start up your container so that it can talk to the other containers of the platform.
You will have to set the $RASA_CORE_TOKEN
environment variable, which you can find
in the settings at http://rasa.examples.com/settings .
#! /bin/bash
echo "copying rasa core model & restarting"
cp -r models/dialogue/* /home/core_project/
sudo docker restart core
echo "stopping and starting app"
sudo docker stop app 2> /dev/null
sudo docker rm app 2> /dev/null
sudo docker run -d -p 5001:5001 \
--name app \
-e RASA_API_ENDPOINT_URL=http://nlu-api:5002 \
-e RASA_REMOTE_CORE_ENDPOINT_URL=http://core:5005 \
-e RASA_CORE_TOKEN=$RASA_CORE_TOKEN \
--link nlu-api \
--link core \
$IMAGE_TAG
Add Some NLU Data¶
Unlike the Rasa Core model, the Rasa NLU model is trained directly on the server.
The training data in the platform should therefore be considered the master copy.
Navigate to the NLU tab in the platform at http://rasa.example.com/inbox, click on Trained Examples
,
and click Upload and Replace
. Upload the training data at data/training_data.json
and hit the train
button.
Try out your bot!¶
Go to http://rasa.example.com/chat - you should see a chat interface similar to the one below.
For more details read Rasa Chat.
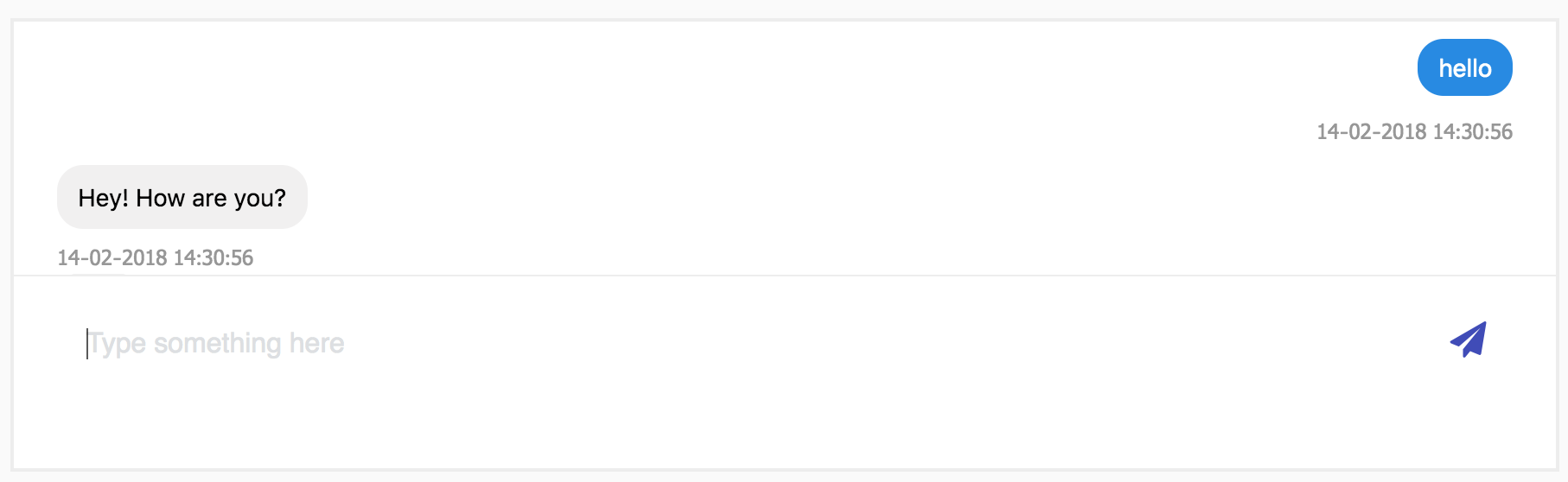
Deploying to a remote server¶
For a CI/CD set up you probably don’t want to build your docker image on the server that’s running your bot.
In the remote
directory there are two scripts, deploy_model.sh
and deploy_app.sh
that show how you
can deploy the application to a remote server. However you will need to set up a docker registry to hold your images.
The easiest option is to create an account at https://hub.docker.com and push your images there.
Deploying a Custom App¶
Note
This tutorial shows you how to run your own app on the rasa platform. Please work through Deploy an Example App before following this tutorial.
Once you’ve got the example app running on your platform deployment, you can make a few changes
Dialogue policies¶
You can make use of any policies provided by Rasa Core. If you want to ship your custom Rasa Core or Rasa NLU code, follow the instructions for Deploying custom Rasa Core or Rasa NLU code.
Choosing an Input Channel¶
The example app is configured to run on RasaChat
, but you
may of course wish to talk to a different channel. To do this,
pass a different Input
object when instantiating
the HttpInputChannel
.
# Facebook Messenger
from rasa_core.channels.facebook import FacebookInput
input_channel = HttpInputChannel(config.self_port, "/", FacebookInput(
fb_verify="changeme",
fb_secret="changeme",
fb_access_token="fb_access_token"))
You can also have both Rasa Chat
and your own channel by simply adding
adding your preferred Input
to the HttpInputChannel
.
# Facebook Messenger
from rasa_core.channels.facebook import FacebookInput
from rasa_extensions.channels.rasa_chat import RasaChatInput
fb_input = FacebookInput(fb_verify="changeme",
fb_secret="changeme",
fb_access_token="fb_access_token")
rasa_in = RasaChatInput(platform_api="https://rasa.example.com/api")
input_channel = HttpInputChannel(config.self_port, "/", fb_input, rasa_in)
Using Other Packages¶
In the example app there is a file called requirements.txt
.
You can add any python packages there which you might need for
executing your actions.
For example, if you wanted to look up an address with the Google Maps API, you would probably want to use the googlemaps python package.
Connecting to the Rasa Core Server¶
The main entrypoint for your application (e.g. main.py
)
should look like this:
import logging
import os
from rasa_core.channels.rest import HttpInputChannel
from rasa_core.remote import RemoteAgent
if __name__ == "__main__":
logging.basicConfig(level="DEBUG")
# instantiate the input channel you want to connect to
from rasa_extensions.core.channels.rasa_chat import RasaChatInput
input_channel = HttpInputChannel(
5001, "/", RasaChatInput(os.environ.get("RASA_API_ENDPOINT_URL")))
agent = RemoteAgent.load('models/dialogue',
os.environ.get("RASA_REMOTE_CORE_ENDPOINT_URL"),
os.environ.get("RASA_CORE_TOKEN"))
agent.handle_channel(input_channel)
In this example, the settings to connect to the remote Rasa Core instance of the platform are read from environment variables:
RASA_REMOTE_CORE_ENDPOINT_URL
: http url to connect to the Core serverRASA_CORE_TOKEN
: To protect the server, requests need to be authenticated with a secure token. This token is stored in/etc/rasaplatform/.env
.
We will see in a minute how these values are set in the docker compose setup.
Create a docker container for your application¶
This is just an example docker file that should guide you in how to create a suitable container for your application. You are free to install any additional required software within this container.
FROM python:2.7-slim
SHELL ["/bin/bash", "-c"]
RUN apt-get update -qq && \
apt-get install -y --no-install-recommends \
build-essential \
wget \
openssh-client \
graphviz-dev \
pkg-config \
git-core \
openssl \
libssl-dev \
libffi6 \
libffi-dev \
libpng12-dev \
curl && \
apt-get clean && \
rm -rf /var/lib/apt/lists/* /tmp/* /var/tmp/* && \
mkdir /app
WORKDIR /app
ARG RASA_PYPI_USER
ARG RASA_PYPI_PASSWORD
# Copy in the python requirements
COPY requirements.txt ./
# we use the Rasa python repository, as there are dependencies that are
# private. any public dependency is still fetched from pypi.python.org
RUN pip install \
-i "https://$RASA_PYPI_USER:$RASA_PYPI_PASSWORD@pypi.rasa.ai/simple/" \
-r requirements.txt
COPY . /app
EXPOSE 5001
CMD ["python", "main.py"]
Deploying that container as part of the Platform¶
To start your container as part of the platform, you need to add it to the
docker-compose
specification. This will tell the docker runner to
start your container as part of the platform.
Instead of changing /etc/rasaplatform/docker-compose.yml
we strongly
recommend to create a file /etc/rasaplatform/docker-compose.override.yml
with your changes. Any changes made to /etc/rasaplatform/docker-compose.yml
might be overwritten by an update.
Here is an example docker-compose.override.yml
that includes the
changes necessary to start the above docker:
version: '3'
services:
app:
build:
context: app
dockerfile: Dockerfile
args:
RASA_PYPI_USER: ${RASA_PYPI_USER}
RASA_PYPI_PASSWORD: ${RASA_PYPI_PASSWORD}
container_name: "app"
environment:
RASA_REMOTE_CORE_ENDPOINT_URL: "http://core:5005"
RASA_CORE_TOKEN: ${RASA_CORE_TOKEN}
RASA_API_ENDPOINT_URL: "http://nlu-api:5002"
expose:
- "5001"
depends_on:
- core
This assumes that your custom application code is stored on the machine
in /etc/rasaplatform/app
and your docker file is located there as well
/etc/rasaplatform/app/Dockerfile
.
After creating this docker-compose.override.yml
, you can use
the following commands to restart the platform with your app:
cd /etc/rasaplatform
sudo docker-compose up --build -d
This setup will build the docker container directly on the server.
Alternatively, you can also build the docker container externally (e.g. on a continuous integration server like travis) and use that image in the docker compose:
version: '3'
services:
app:
image: my-private-docker-registry.com/platform-app:latest
container_name: "app"
environment:
RASA_REMOTE_CORE_ENDPOINT_URL: "http://core:5005"
RASA_CORE_TOKEN: ${RASA_CORE_TOKEN}
RASA_API_ENDPOINT_URL: "http://nlu-api:5002"
expose:
- "5001"
depends_on:
- core
In this case, you need to make sure to pull the image before starting up the server:
cd /etc/rasaplatform
sudo docker login -u USER -p PASSWORD https://my-private-docker-registry.com
sudo docker pull my-private-docker-registry.com/platform-app:latest
After the image is successfully pulled, make sure to login with the Rasa Platform credentials again and restart the platform:
sudo docker login -u _json_key -p "$(cat /etc/rasaplatform/gcr-auth.json)" https://gcr.io
sudo docker-compose pull
sudo docker-compose up -d
Note
sudo docker-compose pull
will also attempt to pull the latest image of your app.
This will fail because you’re logged in with the Rasa credentials, rather than
your own. To update the platform successfully, run
sudo docker-compose pull --ignore-pull-failures
API Keys and other Secrets¶
The safest way to provide secrets to your container is using environment
variables. For example, you can pass an environment variable MY_API_SECRET
in the docker-compose.override.yml
that you can then use in your python
code:
services:
app:
image: my-private-docker-registry.com/platform-app:latest
container_name: "app"
environment:
RASA_REMOTE_CORE_ENDPOINT_URL: "http://core:5005"
RASA_CORE_TOKEN: ${RASA_CORE_TOKEN}
RASA_API_ENDPOINT_URL: "http://nlu-api:5002"
MY_API_SECRET: "secret"
expose:
- "5001"
depends_on:
- core
To access the variable in your python code, use:
import os
os.environ.get("MY_API_SECRET")
Alternatively, you can also copy files containing secrets
into the docker image (provided not many people have access to
the image repository). To do this, add a COPY
command to
your dockerfile:
COPY secrets.txt ./
Architecture Deep Dive¶
The whole platform is dockerized, so we suggest you run your custom code in a docker container as well.
Overview¶
Let’s get started with an overview of the different parts of the Rasa Platform and where your application (e.g. custom actions, in/out channels) fit in:

The Chat User
is the person talking to your bot.
The Platform User
is a user of the management interface to train
and test models.
The image shows the server (docker host) running a number of different containers:
- application code (a docker container running your custom actions and input / output connectors)
- Rasa platform (different apis, the platform ui as well as Core and NLU)
- database
The part you need to provide is highlighted in green. The following instructions are will show you how to create that container.
Creating your application¶
There are a couple of things your application needs to do:
- send and receive messages from the chat channel - the
Input Connector
andOutput Connector
. Your code needs to connect to the chat channel, e.g. for facebook that means starting a webservice, listening for the webhook calls from facebook messenger and sending messages using the facebook API. - execute custom action logic - the
Custom Action Logic
block. This contains any custom action code written, e.g. to validate input or connect to external APIs (see Rasa Core Action docs). - connect to Rasa Core - the
Rasa Core Remote Connector
. For python this implementation is part of the platform python package. If you want to use a different language, you can use the python connector as inspiration. It implements the remote action execution interface from Rasa Core. - upload models to Core / NLU - the green model containers
nlu model
andcore model
. This is not done directly in your application container, instead you upload your stored models to Rasa NLU and Rasa Core.
You can write your application code in any language. We suggest you use python, which will allow you to reuse the Rasa Core Remote Connector as well as existing Input and Output Connectors (like Facebook Messenger).
Throughout the docs, we assume that your application container is started on
the same server as the Platform deployment. On the server, requests to
https://rasa.example.com/app
are automatically forwarded to
port 5001
. If your container is started and listening to that
port it will automatically accept requests from that url. You are free to run
your application container on a different server though.
What’s next?
To make it easier to get started, we created an Deploy an Example App and a small tutorial that guides you through that example.